- HR Brain Pickings
- Posts
- Q&A: People Analytics to Attract, Develop and Retain Talent with Raman Rai
Q&A: People Analytics to Attract, Develop and Retain Talent with Raman Rai
Unlocking the Power of Data: Strategies for Successful Analysis and Distribution
People Analytics is like the cool kid at school who always knows the latest trends and can effortlessly attract a crowd. It's the secret weapon that companies use to attract, develop, and retain top talent, and it's changing the game.
Every week, we schedule our weekly 1:1 with our readers. Want to be featured in an upcoming edition? Click here to introduce yourself.
This week we’re in conversation with Raman Rai, HR and Analytics Leader. We talk about using People Analytics to Attract, Develop and Retain Talent.
The interview is edited for length and clarity.
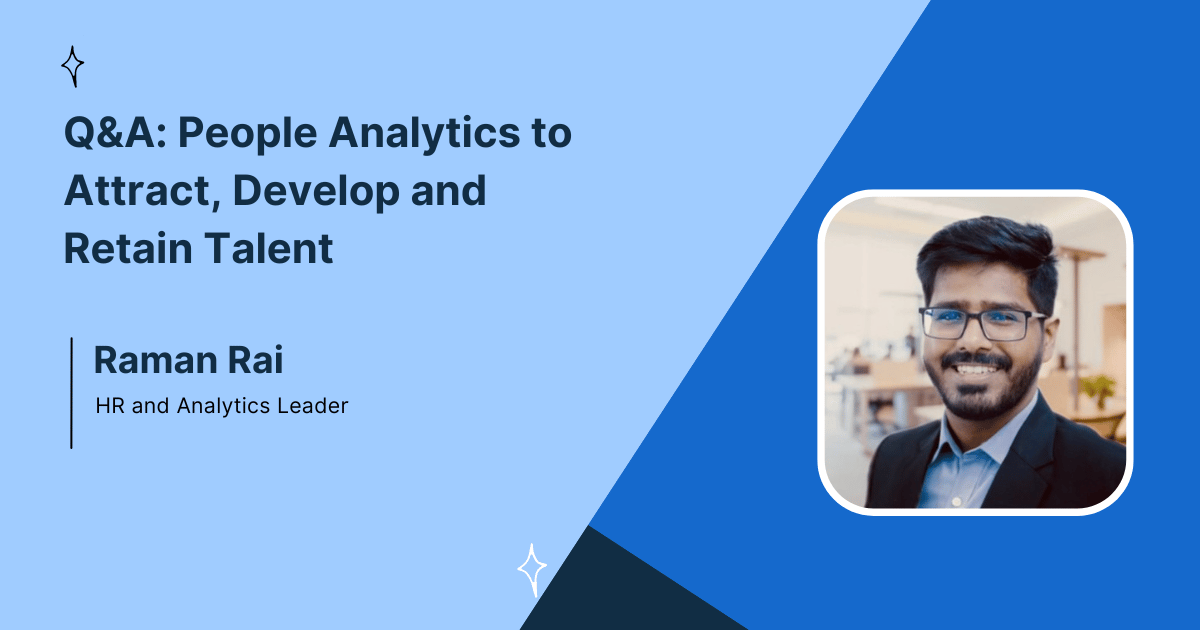
Q- How is People Analytics being used to Attract talent?
People Analytics is a data-driven approach to HR that aims to provide insights into the workforce and guide data-backed decision-making. It involves gathering, analyzing, and interpreting data to optimize business processes and improve employee experience.
People Analytics has three types: descriptive, predictive, and prescriptive. Use cases of all these times cover different touchpoints of the employee lifecycle, such as attraction, retention, engagement, and development.
Descriptive People Analytics:
Descriptive People Analytics is all about analyzing past data to gain insights and understand trends. In the talent attraction stage, descriptive analytics can help organizations identify the best channels of hiring by looking at metrics such as cost-to-hire, time-to-hire, and quality of hire. It can also help organizations understand the speed of hiring, and create diversity slates to ensure equal representation of diverse groups during the hiring process.
Predictive People Analytics:
Predictive People Analytics uses historical data to make predictions about the future. For instance, if a person comes from a particular set of variables, such as a certain type of college, they are likely to stay at an organization for a period between two to three years. They can be used to create models to predict performance by analyzing historical records. During the talent acquisition processes, the data can help organizations decide on the time span for the role they are hiring for.
Prescriptive People Analytics:
Prescriptive People Analytics takes data from predictive analytics a step further by providing actionable insights to optimize business processes. For instance, if predictive analytics predicts that a certain group of employees is likely to leave the organization, prescriptive analytics can help organizations identify the root cause of the problem and recommend solutions to prevent attrition. Prescriptive analytics can also be used to recommend training programs for employees based on their performance history.
Q- How is people analytics used to develop talent?
Moving on to development, we use descriptive analytics to understand the skills of our workforce, as well as performance data to identify high/low performing and high potential employees. This helps us create a performance and potential grid, where we invest more in high-potential but low-performing employees. We also analyze skills data to understand the demand and supply of skills and work with the business to identify the required skills. Startups such as Gloat are providing skill-matching services to help organizations identify talent with the required skills for specific projects. This is known as talent supply chain management, and it's a crucial aspect of development.
Q- How is People analytics used for employee engagement and retention?
This is a highly sophisticated function that is not yet commonly adopted by most companies at a large scale. However, it does exist and some organizations do use it effectively. For example, an AI-powered algorithm can predict the likelihood of an employee, such as John, leaving their job based on various factors like tenure, education level, pedigree, compensation, and compa ratio. If John scores 87 out of 100 on this model, he is considered a high-risk employee, with a score above 80 indicating a high risk.
A prescriptive model can then suggest actions to reduce the score, such as offering a promotion within the next two months. This approach may not be entirely accurate, but it still provides recommendations based on John's profile. Many modern companies in the West have started to use such models, and there are even startups like Humu that do this.
When it comes to engagement level, we have discussed descriptive, predictive, and prescriptive analytics. Moving on to development and performance, descriptive analytics only focuses on skills - what skills does the organization have? However, my biggest challenge with people or HR analytics is the quality of the data. It is either missing or in poor shape, segregated across various platforms, and lacking data hygiene.
Q- What are the possible ways to collect the right data? And what are the mistakes that organizations are making right now in collecting that data?
Challenges in HR Analytics
To harness the power of HR analytics, we need to collect relevant data and analyze it effectively. However, there are several challenges that hinder the progress of HR analytics in many organizations.

Lack of Data Competency
Although technology and data skills have improved in recent years, there is still a long way to go to build a strong data culture in many organizations. Many HR professionals are not comfortable with data, which hinders their ability to use it effectively.
Segregated Systems
The HR tech ecosystem comprises numerous systems that generate and store data. However, these systems are often not integrated, and data is segregated across different platforms. This fragmentation makes it challenging to connect the data through common keys and generate insights. Also, companies may not reconcile the same data collected in different databases, leading to inconsistencies and inaccuracies in data analysis.
Data Quality and Sensitivity
Another issue is data quality, where data may not be clean, consistent, or standardized. For instance, some companies may not collect data at all, while others may collect it in a non-standardized way, making it difficult to analyze. Furthermore, the sensitivity of some HR data may prevent the democratization of data and analysis.
Skills Gap
HR analytics requires a combination of technical and analytical skills, including statistical modeling, machine learning, and data visualization. However, many HR professionals lack these skills, making it challenging to derive insights from data.
Lack of Business Buy-In
Finally, investing in HR analytics requires support and buy-in from business leaders, who may not see the value in data and analysis for HR. Thus, HR professionals need to communicate the benefits of HR analytics and its potential to drive business outcomes effectively.
Q- How do we utilize the data we collect in HR? Are we using it to make decisions, convey it to senior leadership, or communicate it to employees?
Ultimately, we use the data to get a pulse on the company and understand where we're at, what we're doing, and where we're headed.
Once data is recorded and analyzed, the next challenge is to distribute it to business leaders who need to make decisions based on the data. This can be a complex task since these leaders are spread across different levels, geographies, and functions in the business. The solution is to democratize the data, which means making it accessible to everyone who needs it.
Democratizing data requires the implementation of systems, including a central visualization tool that enables data analysis and visualization. The tool should allow role-based access to ensure that each user only accesses the data they are authorized to see. However, ensuring role-based access can be challenging, especially when dealing with complex organizational structures.
Clear dashboards are critical when distributing data to ensure that data points are easy to understand, and role-based access is appropriately established. To create this access, data points need to be categorized, and appropriate access levels need to be determined.
Another challenge when dealing with people is organizational network analytics, including relationship analytics that analyzes related data. For instance, analyzing the social capital score can generate insights into the importance of employees who might not be the best performers in the team. It is essential to retain these employees since they contribute significantly to the team's dynamics.
Moreover, organizational relationship data can also provide insights into diversity and inclusion measures. For example, gender biases can be identified by analyzing communication patterns between male and female managers.
Finally, health data can be analyzed to determine trends in employee communication outside office hours, identifying potential problems in the work culture. This type of information can help leaders identify areas where they can improve the organization's work environment and foster a healthier work-life balance for their employees.
If you want to feature in one of the issues or have a topic we should cover, email Janhavi at [email protected]. Let’s chat!
Reply